“En route” to more equitable urban mobility, thanks to artificial intelligence
Individual cars represent a major source of pollution. But how can you transition from using your own car when you live far from the city center, in an area with little access to public transport? Andrea Araldo, researcher at Télécom SudParis is undertaking a research project that aims to redesign city accessibility, to benefit those excluded from urban mobility.
The transport sector is responsible for 30% of greenhouse gas emissions in France. And when we look more closely, the main culprit appears clearly: individual cars, responsible for over half of the CO2 discharged into the atmosphere by all modes of transport.
To protect the environment, car drivers are therefore thoroughly encouraged to avoid using their car, instead opting for a means of transport that pollutes less. However, this shift is impeded by the uneven distribution of public transport in urban areas. Because while city centers are generally well connected, accessibility proves to be worse on the whole in the suburbs (where walking and waiting times are much longer). This means that personal cars appear to be the only viable option in these areas.
The MuTAS (Multimodal Transit for Accessibility and Sustainability) project, selected by the National Research Agency (ANR) as part of the 2021 general call for projects, aims to reduce these accessibility inequalities at the scale of large cities. The idea is to provide the keys to offering a comprehensive, equitable and multimodal range of mobility options, combining public transport with fixed routes and schedules with on-demand transport services, such as chauffeured cars or rideshares. These modes of transport could pick up where buses and trains leave off in less-connected zones. “In this way, it is a matter of improving accessibility of the suburbs, which would allow residents to leave their personal car in the garage and take public transport, thereby contributing to reducing pollution and congestion on the roads”, says Andrea Araldo, researcher at Télécom SudParis and head of the MuTAS project, but formerly a driving school owner and instructor!
Improving accessibility without sending costs sky-high
But how can on-demand mobility be combined with the range of public transport, without leading to overblown costs for local authorities? The budget issue remains a central challenge for MuTAS. The idea is not to deploy thousands of vehicles on-demand to improve accessibility, but rather to make public transport more equitable within urban areas, for an equivalent cost (or with a limited increase).
This means that many questions must be answered, while respecting this constraint. In which zones should on-demand mobility services be added? How many vehicles need to be deployed? How can these services be adapted to different times throughout the day? And there are also questions regarding public transport. How can bus and train lines be optimized, to efficiently coordinate with on-demand mobility? Which are the best routes to take? Which stations can be eliminated, definitively or only at certain times?
To resolve this complex optimization issue, Araldo and his teams have put forward a strategy using artificial intelligence, in three phases.
Optimizing a graph…
The first involves modeling the problem in the form of a graph. In this graph, the points correspond to bus stops or train stations, with each line represented by a series of arcs, each with a certain trip time. “What must be noted here is that we are only using real-life, public data,” emphasizes Araldo. “Other research has been undertaken around these issues, but at a more abstract level. As part of MuTAS, we are using openly available, standardized data, provided by several cities around the world, including routes, schedules, trip times etc., but also population density statistics. This means we are modeling real public transport systems.” On-demand mobility is also added to the graph in the form of arcs, connecting less accessible areas to points in the network. This translates the idea of allowing residents far from the city center to get to a bus or train station using chauffeured cars or rideshares.
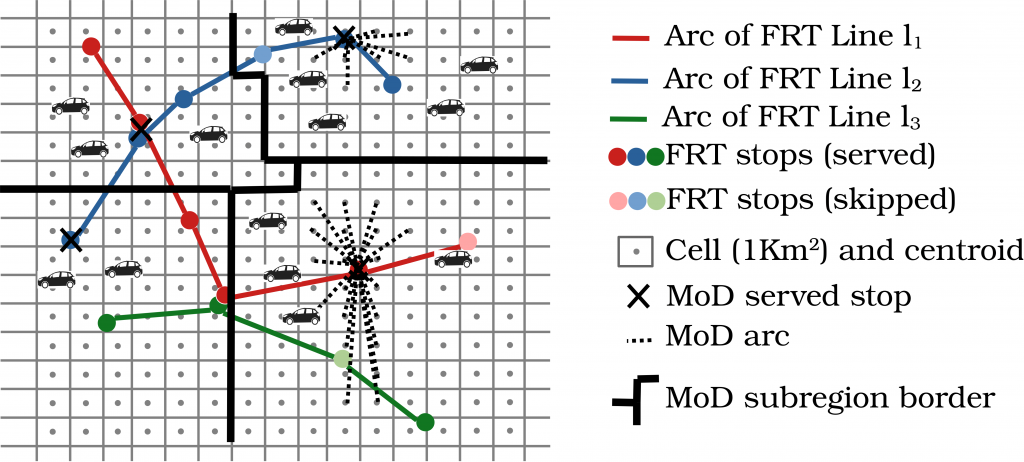
To optimize travel in a certain area, researchers start by modeling public transport lines with a graph.
…using artificial intelligence
This modeled graph acts as the starting point for the second phase. In this phase, a reinforcement learning algorithm is introduced, a method from the field of machine learning. After several iterations, this is what will determine what improvements need to be made to the network, for example, deactivating stations, eliminating lines, adding on-demand mobility services, etc. “Moreover, the system must be capable of adapting its structure dynamically, according to shifts in demand throughout the day,” adds the researcher. “The traditional transport network needs to be dense and extended during peak hours, but it can contract significantly in off-peak hours, with on-demand mobility taking over for the last kilometers, which is more efficient for lower numbers of passengers.”
And that is not the only complex part. Various decisions influence each other: for example, if a bus line is removed from a certain place, more rideshares or chauffeured car services will be needed to replace it. So, the algorithm applies to both public transport and on-demand mobility. The objective will therefore be to reach an optimal situation in terms of equitable distribution of accessibility.
But how can this accessibility be evaluated? There are multiple methods to do so, but researchers have chosen two adapted methods for graph optimization. The first is a ‘velocity score’, corresponding to the maximum distance that can be traveled from a departure point in a limited time (30 minutes for example). The second is a ‘sociality score’, representing the number of people that one can meet from a specific area, also within a limited time.
In concrete terms, the algorithm will take an indicator as a reference, i.e. a measure of the accessibility for the least accessible place in the area. The aim being to make transport options as equitable as possible, it will aim to optimize this indicator (‘max-min’ optimization), while respecting certain restrictions such as cost. To achieve this, it will make a series of decisions concerning the network, initially in a random way. Then, at the end of each iteration, by analyzing the flow of passengers, it will calculate the associated ‘reward’, the improvement in the reference indicator. The algorithm will then stop when the optimum is reached, or else after a pre-determined period.
This approach will allow it to establish knowledge of its environment, associating each network structure (according to the decisions made) with the expected reward. “The advantage of such an approach is that once the algorithm is trained, the knowledge base can be used for another network,” explains Araldo. “For example, I can use the optimization performed for Paris as a starting point for a similar project in Berlin. This represents a precious time-saver compared to traditional methods used to structure transport networks, in which you have to start each new project from zero.”
Testing results on (virtual) users in Ile-de-France
Lastly, the final phase aims to validate the results obtained using a detailed model. While the models from the first phase aim to reproduce reality, they only represent a simplified version. This is important, given that they will then be used for various iterations, as part of the reinforcement learning process. If they had a very high level of detail, the algorithm would require a huge amount of computing power, or too much processing time.
The third phase therefore involves first delicately modeling the transport network in an urban area (in this case, the Ile-de-France region), still using real-life data, but more detailed this time. To integrate all this information, researchers use a simulator called SimMobility, developed at MIT in a project to which Araldo contributed. The tool makes it possible to simulate the behavior of populations at an individual level, each person represented by an ‘agent’ with their own characteristics and preferences (activities planned during the days, trips to take, desire to reduce walking time or minimize number of changes, etc.). It was based on the work of Daniel McFadden (Nobel Prize for Economics in 2000) and Moshe Ben-Akiva on ‘discrete choice models’, which makes it possible to predict choices between multiple modes of transport.
With the help of this simulator and public databases (socio-demographic studies, road networks, numbers of passengers, etc.), Araldo and his team, in collaboration with MIT, will generate a synthetic population, representing Ile-de-France users, with a calibration phase. Once the model faithfully reproduces reality, it will be possible to submit it to the new optimized transport system and simulate user reactions. “It is important to always remember that it’s only a simulation,” reminds the researcher. “While our approach allows us to realistically predict user behavior, it certainly does not correspond 100% to reality. To get closer, more detailed analysis and deeper collaborations with transport management bodies will be needed.”
Nevertheless, results obtained could serve to support more equitable urban mobility and in time, reduce its environmental footprint. Especially since the rise of electric vehicles and automation could increase the environmental benefits. However, according to Araldo, “electric, self-driving cars do not represent a miracle solution to save the planet. They will only prove to be a truly eco-friendly option as part of a multimodal public transport network.”
Bastien Contreras