Decision support tools for maritime accident management
The European MANIFESTS project, launched in January, is a two-year project bringing together a consortium of nine research institutions and public administrations with complementary expertise in managing maritime accidents. Funded by the European Commission, this project aims to improve responses to emergencies related to these accidents. An interview with Laurent Aprin, a researcher at IMT Mines Alès, a project partner.
Could you describe the broader context of the MANIFESTS project?
Laurent Aprin –The MANIFESTS project (Managing Risks and Impacts From Evaporating and Gaseous Substances to Population Safety) is a follow-up to the European HNS-MS project funded from 2015 to 2017 by the European Commission’s Directorate General for European Civil Protection and Humanitarian Aid (DG-ECHO). The purpose of this project was to study and model the consequences of chemical spills in the ocean and determine the vulnerability of the environment, people and goods depending on the chemicals spilled. We wanted to continue our research by expanding the consortium and addressing questions submitted by the various stakeholders at the end-of-project meeting, in particular the consequences of evaporating substances that are likely to form toxic clouds, which are flammable, or even explosive.
What is the aim of the MANIFESTS project?
LA – Responding to maritime accidents can be especially challenging when they involve Hazardous and Noxious Substances (HNS) which act like gases or evaporators. Due to their potential to form toxic or combustible clouds, fact-based decisions are needed to protect the crew, responders, coastal communities and the environment. But when an accident is declared, key information for assessing risks for responders or emergency teams is not always available. Allowing a ship that presents a risk to dock in a place of refuge due to a lack of knowledge and data could have major implications for coastal communities. The aim of MANIFESTS is to respond to these uncertainties and improve response capacity with decision support tools and novel and innovative operational guidelines. How so? By facilitating access to knowledge and databases, all of which are hosted on a dedicated open source web platform accessible to planners and responders.
How will you achieve this goal?
LA – The MANIFESTS project is divided into four activities (workpackages, WP) supported by two cross-project activities, project management (WP1) and project communication (WP6). The technical work includes producing new data and knowledge on gases and evaporating substances that may be released during marine accidents. This information will be obtained by acquiring knowledge from the literature and research data (WP2). WP3 involves developing methods to assess and manage risks and testing response tools through computer-based and field trials. WP4 will focus on developing and improving tools for modeling HNS behavior and developing a MANIFESTS decision support system. This WP includes developing new tools based on the previously described WPs and upgrading the models developed in the existing HNS-MS and MARINER projects (WP5).
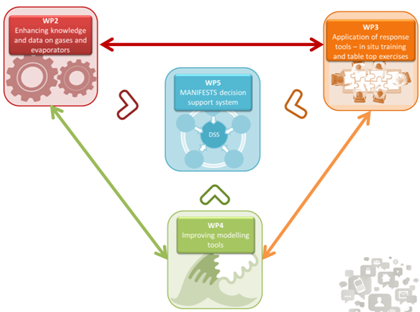
What scientific expertise are IMT Mines Alès researchers bringing to this project?
LA – IMT Mines Alès[1] researchers are primarily involved in two WPs:
- WP2: improving knowledge and data on gases and evaporating substances for which IMT Mines Alès is the coordinator. This task aims to characterize and theoretically and experimentally assess the behavior and impacts of HNS when they are released into the ocean, with a particular focus on the release of volatile substances that may lead to the formation of a potentially toxic, flammable and/or explosive gas cloud.
- WP6: strategy for dissemination, exploitation and visibility, in particular to develop proof of concept (PoC) for a serious games to train emergency responders and planners involved in managing marine pollution events. Using an immersive scenario, this crisis simulation makes it possible to test the implementation of response plans, the response cell’s capacity to fulfill its missions (including adapting during a dynamically evolving scenario) and to make defensible decisions under demanding, realistic conditions.
Who are your partners for this project and how are you working together?
LA – The project consortium is coordinated by Cedre (France)[2], and includes 9 research institutions and public administrations from 6 countries (France, Belgium, UK, Norway, Spain, Portugal) with strong complementary expertise: ARMINES/IMT Mines Alès (France), Royal Belgium Institute of Natural Science (RBINS, Belgium), Instituto Tecnológico para el Control del Medio Marino de Galicia (INTECMAR, Spain), Centro tecnologico del mar/Fundacion CETMAR (Spain), Instituto superior tecnico (Portugal), Department of Health (UK), Meteorologisk Institutt (Norway) and the Federal Public Service for Public Health, Food Chain Safety and Environment (Belgium). They are involved in all the aspects of marine pollution addressed by the project: chemical analysis, pollution modeling, developing decision support tools, risk assessment and management, training and exercises, knowledge transfer. MANIFESTS will also benefit from collaboration with an advisory committee comprising 6 national maritime authorities who will be the primary end-users of the project results, including the French Navy, CEPPOL (Centre of Practical Expertise for Pollution Response) and customs for France.
What are the next big steps for the project?
LA – The MANIFESTS project was launched on 1 January 20201 and is set to run for two years. The first phase will involve an accident study and a literature review of the modeling of the behavior of evaporating substances in the ocean. The next steps will focus on creating experimental designs to characterize the evaporation rate of substances and the consequences of explosions, programming consequence models (dispersion, fire and explosion) and conducting a large-scale trial in the Atlantic Ocean.
[1] The IMT Mines Alès team includes Laurent Aprin, Aurélia Bony-Dandrieux, Philippe Bouillet, Frédéric Heymes, Christian Lopez and Jérôme Tixier.
[2] Laura Cotte, engineer, and Stéphane Le Floch, Head of the Research Department at the Centre for Documentation, Research and Experimentation on Accidental Water Pollution (Cedre), are the initiators and coordinators of the project.
Interview by Véronique Charlet