Sand, an increasingly scarce resource that needs to be replaced
Humans are big consumers of sand, to the extent that this now valuable resource is becoming increasingly scarce. Being in such high demand, it is extracted in conditions that aren’t always respectful of the environment. With the increasing scarcity of sand and the sometimes devastating consequences of mining at beaches, it is becoming crucial to find alternatives. Isabelle Cojan and Nor-Edine Abriak, researchers in geoscience and geomaterials at Mines ParisTech and IMT Lille Douai respectively, explain the stakes involved with regards this resource.
“After air and water, sand is the third most essential resource for human beings” explains Isabelle Cojan, researcher in geoscience at Mines ParisTech. Sand is, of course, used indirectly by most of us, since the major part of this resource is consumed by the construction industry. 15 to 20 billion tons of sand are used every year in the construction of buildings and roads all over the world and in land reclamation. In comparison, the amount used in other applications, such as micro-computing or glass, detergent and cosmetics manufacturing, is around 0.2 billion tons. On an individual scale, global sand consumption stands at 18 kg per person per day.
At first glance, our planet could easily provide the enormous amount of sand required by humanity. A quarter of the surface of the Earth’s continents is covered by huge deserts of which nearly 20% of the surface is occupied by dune fields. The problem is that this aeolian sand is unusable for construction or reclamation: “The grains of desert sand are too smooth, too fine, and cannot bind with the cements to make concrete,” explains Isabelle Cojan. For other reasons, including economic ones, marine sands can only be exploited at shallow depths, as is currently the case in the North Sea. The volume of the Earth’s available sand is significantly smaller once these areas are removed from the equation.
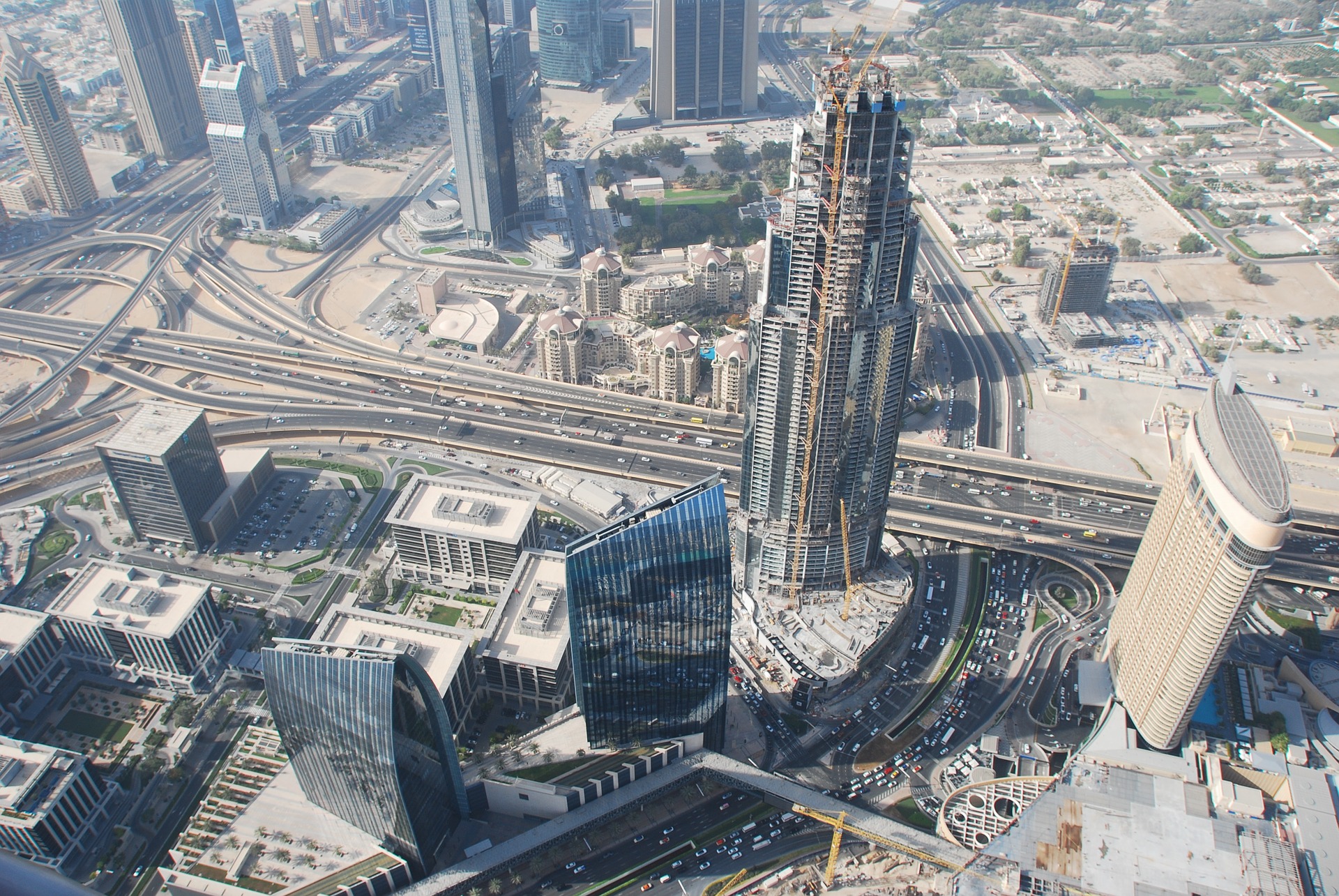
Deserts are poor suppliers of construction sand. The United Arab Emirates is therefore paradoxically forced to import sand to support the development of its capital, Dubai, which is located just a stone’s throw from the immense desert of the Arabian Peninsula.
Deserts are poor suppliers of construction sand. The United Arab Emirates is therefore paradoxically forced to import sand to support the development of its capital, Dubai, which is located just a stone’s throw from the immense desert of the Arabian Peninsula.
So where do we find this precious sediment? Silica-rich sands, such as those found under the Fontainebleau forest, are reserved for the production of glass and silicon. On the other hand, the exploitation of fossil deposits is limited by the anthropization of certain regions, which makes it difficult to open quarries. For the construction industry, the sands of rivers and coastal deposits fed partly by river flow must be used. The grains of alluvium from beaches are angular enough to cling to cements. Although the quantities of sand on beaches may seem huge to the human eye, they are not really that big. In fact, most of the sediments in the alluvial plains of our rivers and coastlines are inherited from the large-scale erosion that occurred during the Quaternary Ice Age. Today, with building developments along riverbanks and less erosion, Isabelle Cojan points out that “we consume twice as much sand as the amount the rivers bring to the coastal regions.”
Dams not only retain water, they also prevent sediment from descending downstream. The researcher at Mines ParisTech points to the example of the watercourse of the Durance, a tributary of the Rhône that she studied: “Before development, it deposited 3 million tons of sediment in the Mediterranean every year. Today, this quantity is between 0.1 and 0.5 million tons.” Most of the sediment provided by the erosion of the watershed is trapped by infrastructure, and settles to the bottom of artificial water reservoirs.
The sand rush and its environmental impact
As a result of the lower release of sediment into the seas and oceans, some beaches are naturally shrinking, especially where the extraction industry takes sand from the coasts. Countries are thus in a critical situation where entire beaches are disappearing. This is the case in Togo, for example, and several other African countries where sand is extracted without too many legislative constraints. “The Comoros derives a lot of its income from tourism, and is extracting more and more sand from beaches to support its economic development because there is no significant reserve of sand elsewhere on the islands,” explains Isabelle Cojan. The extraction of large volumes of sand leads to coastal erosion and land receding to the sea. The situation is similar in other parts of the world. “Singapore has significantly increased its surface area by developing polders in the sea,” the researcher continues. “Nearby islands at the surface of the water providing an easy supply of sand disappeared before the regulations of the countries concerned prohibited such extraction.”
In Europe, practices are more closely controlled. In France, in particular, extraction from riverbeds – a practice which dates back thousands of years – was carried out until the 1970s. The poorly regulated exploitation of alluvial deposits led to changes in river profiles, leading to the scouring of bridge anchorages, which then became fragile and sometimes even collapsed. Extraction from rivers has since been forbidden, and only deposits on alluvial plains that do not directly impact riverbeds may be used for extraction. On the coast, exploitation is regulated by the French Environmental Code and Mining Code, which prohibit any extraction that could directly or indirectly compromise the integrity of a beach.
However, despite this legislation, indirect adverse consequences are difficult to prevent. The impact of sand extraction in the medium term is complex for researchers to model. “In coastal areas, we have to take account of a set of complex processes linked to tides, storms, coastal drift, vegetation cover, tourism and port facilities“ lists Isabelle Cojan. In some cases, sustainable extraction entails no danger. In other situations, a slight change in the profile of the beach could have serious consequences. “This can lead to a significant retreat of the coastline during storms and flooding of the hinterland by marine waters, especially during equinox storms,” the researcher continues. On coastline that is undergoing natural erosion with low-relief hinterlands, sand extraction may, over time, lead to an irreversible destabilization of the coast.
The implications of the disappearance of beaches are not only aesthetic. Beaches and the dunes that very often lie along their edge constitute a natural sedimentary barrier against the onslaught of the waves. They are the primary and most important source of protection against erosion. Beaches limit, for example, the retreat of chalk cliffs. On coasts with low landforms, beach-dune systems form a barrier against the entry of the sea into the land. When water breaches the natural sediment barrier, salinization of fields can occur leading to a drastic change in farming conditions and can ruin arable land.
What are the alternatives to sand?
Faced with the scarcity of this resource, new avenues are being explored to find an alternative to sand. Recycled concrete, glass or metallurgical waste can be used to replace sediment in the composition of concrete. However, building materials produced in this way encounter performance problems: “They age very quickly and can release pollutants over time,” explains Isabelle Cojan. Another limitation is that these alternative options are currently not sufficient in volume. France produces 370 million tons of sand annually, whereas recycling only produces 20 million tons.
Also read on I’MTech Recycling concrete and sediment to create new materials
Major efforts to structure a dedicated recycling sector would be necessary, with all the economic and political debates at national and local levels that this implies. Since manufacturers want high-performance products, this could not be done until research has found a way to limit the aging and pollution of materials made from recycled materials. While recycling should not be ruled out, it is clear that the solution is only feasible over a relatively long time scale.
In the shorter term, another alternative could come from the use of other sand deposits currently considered as waste. Through pioneering work in geomaterials at IMT Lille Douai, Nor-Edine Abriak has demonstrated that it is possible to exploit dredging sand. This sediment comes from the bottom of rivers and streams, and is extracted for water course development. Dredging is mainly used to allow waterway navigation and large quantities of sand are extracted every year from ports and river mouths. “When I started my research on the subject a few years ago, the port of Dunkirk was very congested with sediments,” recalls the researcher. He joined forces with the local authorities to set up a research chair called Ecosed in order to find a way to use this sand.
For the construction industry, the major drawback of dredging sediments is their high content in clay particles. “Clay is a nightmare for people working with concrete,” warns Nor-Edine Abriak. “The particles can swell, increasing the setting time of the cement in the concrete and potentially diminishing the performance of the final material.” It is customary to use a sieve to separate the clay, which requires equipment and dedicated logistics, and therefore additional costs. For this reason, these sediments are rejected by industrial players. “The only way to be competitive with these sediments is to be able to use them as they are, without a separation process,” admits the leader of the Ecosed Chair. The research at IMT Lille Douai has led to a more convenient and rapid treatment process using lime that eliminates the need for sieving. This process also breaks down the organic matter in the sediments and improves the setting of the cement, making it possible to rapidly use the sand extracted from the bottom of the port of Dunkirk.
The Ecosed Chair has also provided a way to overcome another problem, that of the salinity of these shallow sands in contact with seawater. Salt corrodes materials, therefore shortening the useful life of concrete. To remove it, the researchers used a simple water wash. “We showed that the dredging sand could simply be stored in large lagoons and the salt would be drained by the rain,” explains Nor-Edine Abriak. The solution is a simple one, provided there is enough space nearby to build the lagoons, which is the case in the area around Dunkirk.
With these results, the team of scientists demonstrated that dredging sediments extracted from the bottom of ports could be used as an alternative to beach sediments, instead of being considered as waste. “We were the first in the world to prove this was possible,” Nor-Edine Abriak says proudly. This solution does not provide a full replacement, as dredged sediments have different mechanical properties that must be taken into account in order not to affect the durability of the materials. The first scaling tests showed that dredging sands could be used in proportions of up to 70% in the composition of materials for roads and 12% for buildings, with no loss of quality for the end material.
Because almost all ports have to carry out dredging, this research offers a major opportunity to reduce sand extraction from beaches and alluvium. In early March, the Ecosed team went to Morocco to launch a second chair on waste recovery and dredging sands in particular: “the same situation as in Dunkirk can be seen in Tangier and Agadir,” explains the researcher, highlighting the global nature of the problem. In June 2019, the Ecosed Chair in France became Ecosed Digital 4.0, going from a budget of €2 million to €24 million with the aim of structuring a specific sector for the recovery of dredged sediments in France. While this work alone will not fully solve the problem of sand scarcity, it will nevertheless start an impetus to reduce sand extraction in areas where such mining is threatening. It must also be ensured that this type of initiative is scaled up, both nationally and internationally.